The Intersection Of Machine Learning And Embedded Systems A
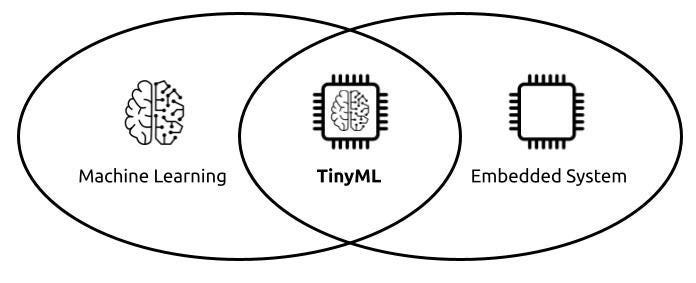
The Intersection Of Machine Learning And Embedded Systems A The intersection of machine learning and embedded systems the intersection of machine learning and embedded systems offers a novel paradigm, often referred to as ‘edge computing’ or ‘edge ai. 1️⃣ model selection and training. choosing the right ml model for embedded use involves balancing accuracy with efficiency. models must be trained to handle the specific tasks they’ll perform on the embedded system. training can occur on the cloud or on device, depending on the complexity and data requirements.
1 Artificial Intelligence The Intersection Of Machine Learning Deep As machine learning in embedded systems is covering more applications and growing in demand, hardware design companies are focusing their efforts on developing ml optimized chips. in fact, the ai chip market is estimated to grow at a cagr of 40.5% by 2030, reaching a worth of $227.6 billion , with nvidia, intel, and google investing heavily in. Ese 3600: tiny ml. embedded machine learning is a cutting edge field that brings the transformative power of machine learning (ml) to the performance constrained and power constrained domain of embedded systems to develop useful and exciting internet of things solutions. this is an introductory course at the intersection of machine learning (ml. This course provides a foundation for you to understand this emerging field. tinyml is at the intersection of embedded machine learning (ml) applications, algorithms, hardware, and software. tinyml differs from mainstream machine learning (e.g., server and cloud) in that it requires not only software expertise, but also embedded hardware expertise. Tiny machine learning (tinyml) is a burgeoning field at the intersection of embedded systems and machine learning. the world has over 250 billion microcontrollers (ic insights, 2020), with strong growth projected over coming years. as such, a new range of embedded applications are emerging for neural networks. because these models are extremely.
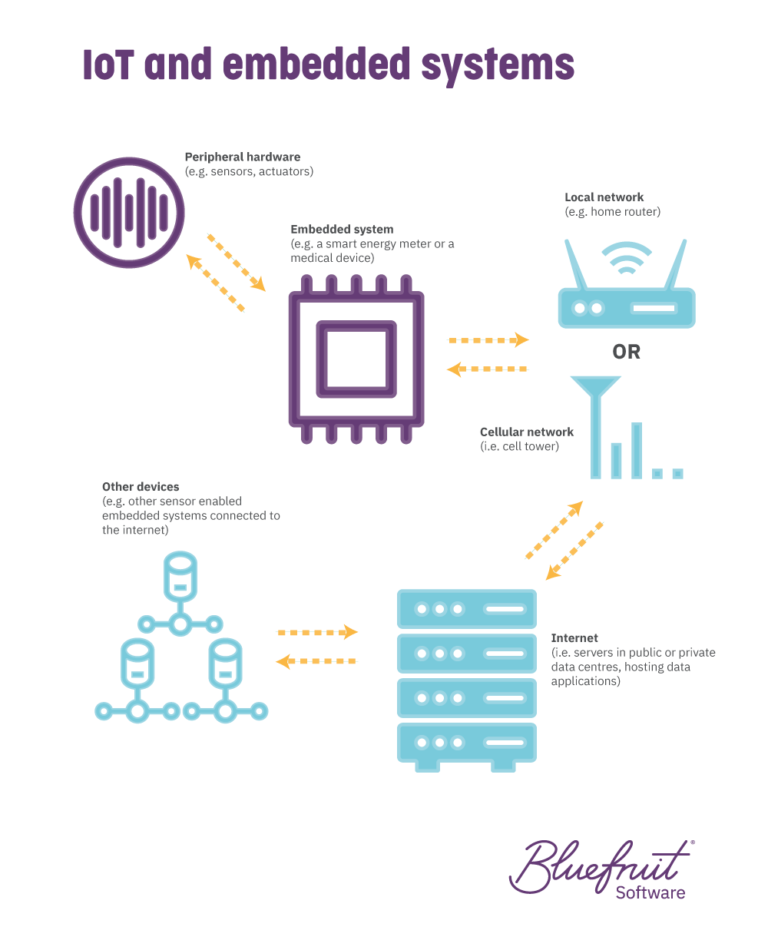
Machine Learning Embedded Systems Exploring The Relationship This course provides a foundation for you to understand this emerging field. tinyml is at the intersection of embedded machine learning (ml) applications, algorithms, hardware, and software. tinyml differs from mainstream machine learning (e.g., server and cloud) in that it requires not only software expertise, but also embedded hardware expertise. Tiny machine learning (tinyml) is a burgeoning field at the intersection of embedded systems and machine learning. the world has over 250 billion microcontrollers (ic insights, 2020), with strong growth projected over coming years. as such, a new range of embedded applications are emerging for neural networks. because these models are extremely. Tinyml sits at the intersection of embedded systems and machine learning, and is targeted at enabling new embedded device applications (figure 2). tinyml focuses on deploying simple yet powerful models onto microcontrollers at the network edge. In perceptron: the inputs can be real numbers; (including threshold) can be learned trained. the weights. in perceptron: like mp neuron, the perceptron separates the input space into two halves. however, all inputs producing 1 lie on one side, and. those producing 0 lie on the other side.

The Benefits And Techniques Of Machine Learning In Embedded Systems Tinyml sits at the intersection of embedded systems and machine learning, and is targeted at enabling new embedded device applications (figure 2). tinyml focuses on deploying simple yet powerful models onto microcontrollers at the network edge. In perceptron: the inputs can be real numbers; (including threshold) can be learned trained. the weights. in perceptron: like mp neuron, the perceptron separates the input space into two halves. however, all inputs producing 1 lie on one side, and. those producing 0 lie on the other side.
Exploring The Intersection Of Embedded Systems And Vlsi Design
Comments are closed.