Peter Buhlmann 5 November 2020

Peter Buhlmann 5 November 2020 Youtube Topic: invariance, causality and robustness in statistical learningabstract: interpretable and reliable machine learning and artificial intelligence is a big. 2002. estimating high dimensional directed acyclic graphs with the pc algorithm. m kalisch, p bühlman. journal of machine learning research 8 (3) , 2007. 1093. 2007. causal inference by using invariant prediction: identification and confidence intervals. j peters, p bühlmann, n meinshausen.
Peter Bгјhlmann Wird Neuer Nab Prг Sident вђ Zudem Drei Neue 1 code implementation • 5 nov 2019 • niklas pfister, evan g. williams, jonas peters, ruedi aebersold, peter bühlmann in particular, it is useful to distinguish between stable and unstable predictors, i. e., predictors which have a fixed or a changing functional dependence on the response, respectively. Peter bÜhlmann | cited by 33,149 | of eth zurich, zürich (eth zürich) | read 369 publications | contact peter bÜhlmann. Assigning statistical uncertainties, stability and robustness are potentially useful to (partially) improve the situation algorithms, machine learning and scientific discoveries. we should aim for. better replicability stability robustness and better interpretation. mosteller and tukey (1968): “one hallmark of the statistically conscious. Peter bühlmann is professor, seminar for statistics, eth zürich, rämistrasse 101, ch 8092 zürich, switzerland (e mail: [email protected]). 1discussed in 10.1214 20 sts768, 10.1214 20 sts772; rejoinder at 10.1214 20 sts797. 2this article is covering material of the 2018 neyman lecture but also describes new developments in section 5.
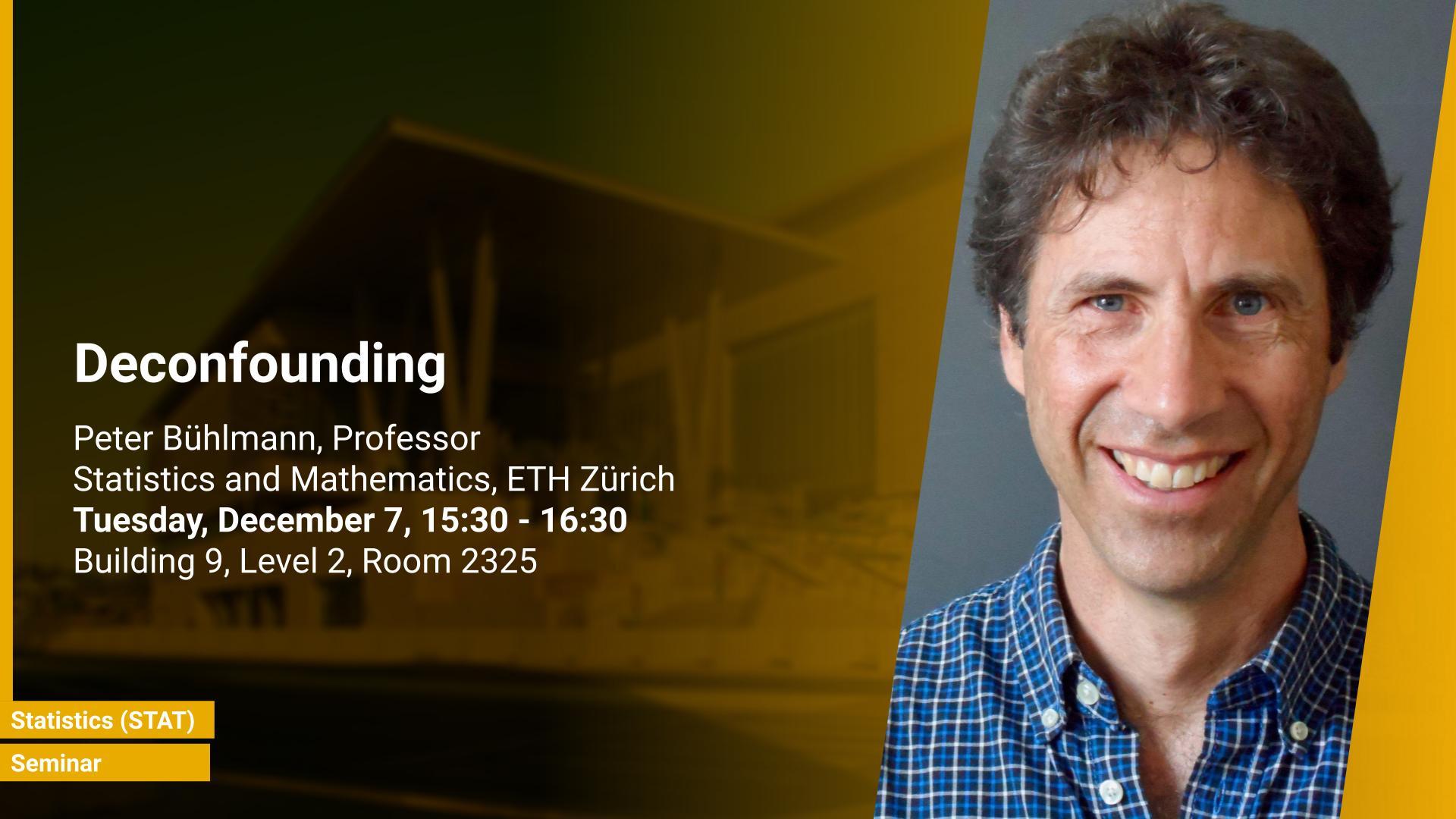
Deconfounding Stat Statistics Assigning statistical uncertainties, stability and robustness are potentially useful to (partially) improve the situation algorithms, machine learning and scientific discoveries. we should aim for. better replicability stability robustness and better interpretation. mosteller and tukey (1968): “one hallmark of the statistically conscious. Peter bühlmann is professor, seminar for statistics, eth zürich, rämistrasse 101, ch 8092 zürich, switzerland (e mail: [email protected]). 1discussed in 10.1214 20 sts768, 10.1214 20 sts772; rejoinder at 10.1214 20 sts797. 2this article is covering material of the 2018 neyman lecture but also describes new developments in section 5. Bühlmann, p. and van de geer, s. (2011). statistics for high dimensional data: methods, theory and applications. springer. statistical analysis for high dimensional data. the a. Peter bühlmann is professor of statistics, seminar for statistics, eth zürich, rämistrasse 101, ch 8092 zürich, switzerland (e mail: [email protected]). then ols a(γ = 0) is adjusting for these first princi pal components and aims to remove hidden confounding bias in estimating the causal parameter β. this common.
Stream Spidserne Peter Chr Bгјhlmann Fra Tv2 Nord Lige Her Bühlmann, p. and van de geer, s. (2011). statistics for high dimensional data: methods, theory and applications. springer. statistical analysis for high dimensional data. the a. Peter bühlmann is professor of statistics, seminar for statistics, eth zürich, rämistrasse 101, ch 8092 zürich, switzerland (e mail: [email protected]). then ols a(γ = 0) is adjusting for these first princi pal components and aims to remove hidden confounding bias in estimating the causal parameter β. this common.

Science Stories Interview With Peter Bгјhlmann вђ Department Of
Comments are closed.