Github Lnrobertson Customer Segmentation 3 Years Of Hotel Data Will
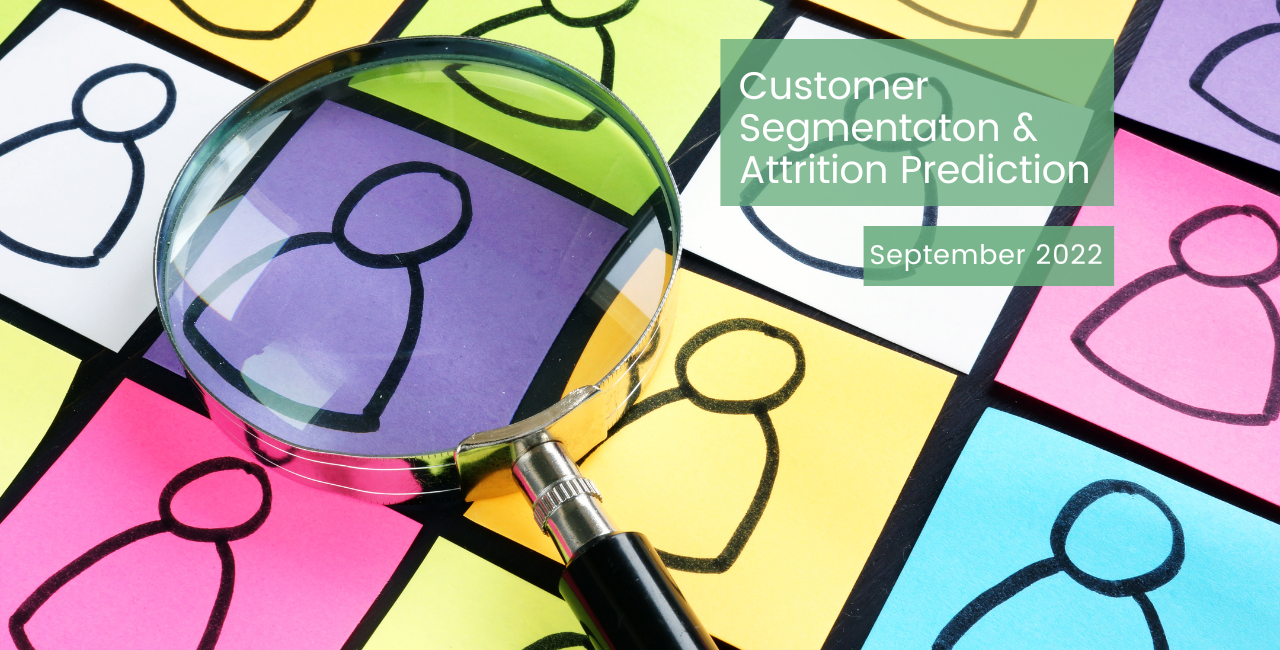
Github Lnrobertson Customer Segmentation 3 Years Of Hotel Data Will A real world customer dataset with 31 variables describes 83,590 instances (customers) from a hotel in lisbon, portugal. instances include; customer personal, behavioral, demographic, and geographical information for 3 full years. See my customer attrition prediction project where i used clustering segmentation and classification on a dataset with 3 years of customer behavior data. š» checkout my beer ratings prediction repo where i used hierarchical clustering and linear regression on a dataset from beeradvocate.
Github Sid2605 Hotel Customer Segmentation Add this topic to your repo. to associate your repository with the customer segmentation analysis topic, visit your repo's landing page and select "manage topics." github is where people build software. more than 100 million people use github to discover, fork, and contribute to over 420 million projects. Intro. a customer segmentation project can be approached in multiple ways. in this article i will teach you advanced techniques, not only to define the clusters, but to analyze the result. this post is intended for those data scientists who want to have several tools to address clustering problems and be one step closer to being seniors ds. Letās group the customers by clusters and see the average values for each variable. df segm analysis = df std.groupby(['segment k means']).mean() df segm analysis. itās time to interpret our. February 6, 2021. tl;dr: a data science tutorial on using k means and decision trees together. customer segmentation (sometimes called market segmentation) is ubiqutous in the private sector. we think about bucketing people into k k mutually exclusive and collectively exhausting (mece) groups. the premise being that instead of having 1 strategy.
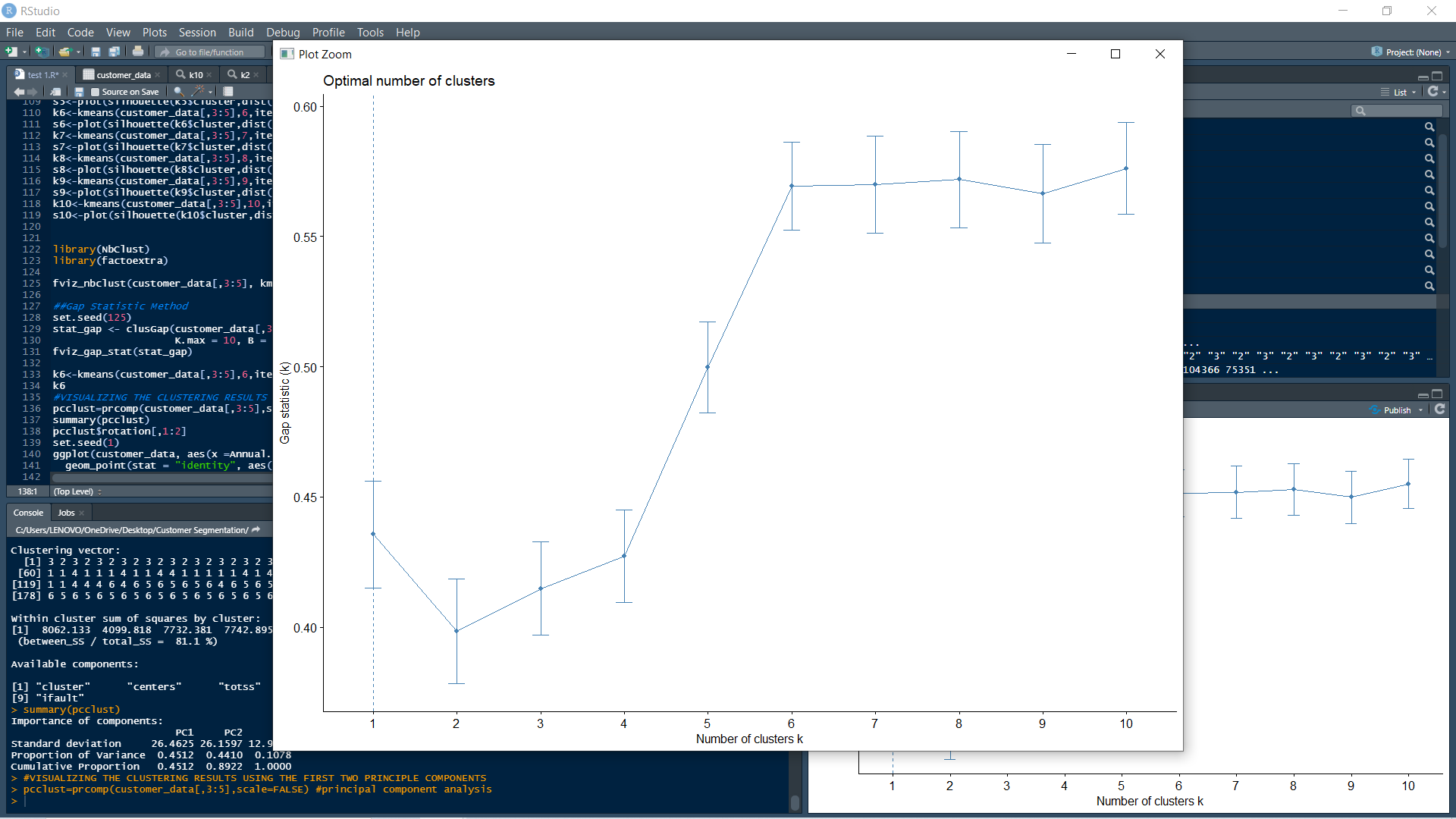
Github Anushka7310 Customer Segmentation Customer Segmentation Letās group the customers by clusters and see the average values for each variable. df segm analysis = df std.groupby(['segment k means']).mean() df segm analysis. itās time to interpret our. February 6, 2021. tl;dr: a data science tutorial on using k means and decision trees together. customer segmentation (sometimes called market segmentation) is ubiqutous in the private sector. we think about bucketing people into k k mutually exclusive and collectively exhausting (mece) groups. the premise being that instead of having 1 strategy. K means tries to address two questions: (1) k: the number of clusters (groups) we expect to find in the dataset and (2) means: the average distance of data to each cluster center (centroid) which we try to minimize. also, one thing of note is that k means comes with several variations, typically :. While i cannot stop customers from canceling their reservation, i can help hotels predict if a new booking will be canceled or not in order to manage their business accordingly and increase their revenue. the dataset i found provided real life booking data from two hotels: a resort in the algarve region (h1) and a city hotel (h2) in lisbon.
Comments are closed.